Viewpoint: Revolutionising nuclear safety documentation
.jpg)
"The nuclear industry is underpinned by its documentation - authoring, verifying and modifying these documents are critical to enable the safe operation of its nuclear power plants. However, simply because the established processes in document production and reviewing have been the same for the last 40+ years, it does not mean they're flawless.
Existing processes can be extremely lengthy and costly (documents can take more than a year to be approved). Therefore, identifying and decoding links between systems or system boundaries from our existing documents is hard unless you're an expert.
However, we can now create artificial intelligence (AI) solutions that can automate and enhance manual processes; these include reading and extracting information from technical nuclear documents, which in turn could provide both a holistic and detailed view of a power plant.
What do we mean by AI solution?
When combining an industry that is riddled with acronyms with one that is full of buzzwords, it's easy to get lost in the volume of individual capital letters. The following section attempts to demystify some of these modern terms, often overused but seldom explained.
The key terms to know are:
Machine Learning (ML) - whose models iteratively learn from data and can find hidden insights without being told where to look.
Natural Language Processing (NLP) - whose techniques are used to analyse and process unstructured text and audio.
By combining ML and NLP, we can start answering questions that previously would have needed the input of subject matter experts. We may think that our "experience" means we understand these documents better, but we are in fact training our own "biological machine learning model" to look for key terms or recognise specific descriptions. If we think about how we recognise dogs - we don't need to know every dog breed to correctly identify whether an animal is a dog.
However, not all models need to be "trained" to start achieving operational benefits. There are a variety of established techniques that can help automate manual activities with minimal computational cost. These can automatically ingest, process and extract information (e.g. key systems or hazards) from thousands of documents much faster than even an experienced engineer could.
How do these techniques work?
In short, humans look at words, and machines look at numbers. NLP models translate words, sentences and documents into vectors to create structure out of the inherently unstructured natural language data. This means we can develop solutions that don't simply look at relative term frequency to determine its importance. Instead, these can consider other factors, such as the context of surrounding words on the meaning of individual words. We can then use these distributed numerical representations of word features to mathematically (e.g. cosine similarity between vectors) detect similarities between words, sentences and documents.
Using these modern techniques, we could:
Subsequently, enable us to:
• Train our algorithms to recognise systems from its descriptions, safety claims, or operations without the name of the system needing to be explicitly mentioned.
• Provide interactive visualisations to filter and drill down to key parameters (e.g. identifying all relevant documents and information associated with the Irradiated Fuel Dismantling Facility).
• Have a solution that is always up to date and can look at increasing numbers of documents without significant additional cost.
• Reduce the number of documents needing detailed examination and the volume of information stored on localised spreadsheets.
• Increase accessibility to all personnel working within the organisation, helping to train junior engineers who need to learn about key systems.
Can these models make mistakes?
Yes, but so can we. These solutions help us tackle the repetitive or time-consuming tasks (that are currently unreachable) more effectively. By setting up this data-driven process coupled with clear performance measurements, we can develop a robust methodology. In turn, this can help engineers answer even the more in-depth questions. For instance, what are the implications on the flask hall crane's existing safety claims if we double the frequency of operations?
These techniques mean that the documents are no longer just the items you read to answer a specific question but become the building blocks of a network. This could enable a solution which ingests new assessments (e.g. updated metrics on the degradation of ferritic steel caused by nuclear irradiation), automatically extracts key values (e.g. fracture toughness), identifies all relevant systems impacted and subsequently runs quantitative assessments using these updated metrics to determine the corresponding implications on existing safety claims.
So, where next?
These solutions are there to support and enhance the engineers; not replace them. As the volume of data keeps rising, we can't afford to be sceptical about what are now established methods. If we do not start leveraging these techniques to help our engineers today, our workforce, not our physical systems, may become tomorrow's single point of failure.
Until our industry takes its first step into embracing innovation, we will never know the full extent of what AI can do in the nuclear industry. By starting small and tackling specific challenges we can build solutions that have an impact relatively quickly. Whilst there is more work to be done before we can develop AI solutions that can generate our safety cases by themselves, there is a lot we can do today that will bring tangible benefits."
Julianne Antrobus is Global Head of Nuclear, and Derreck Van Gelderen is a Data and AI expert at PA Consulting.
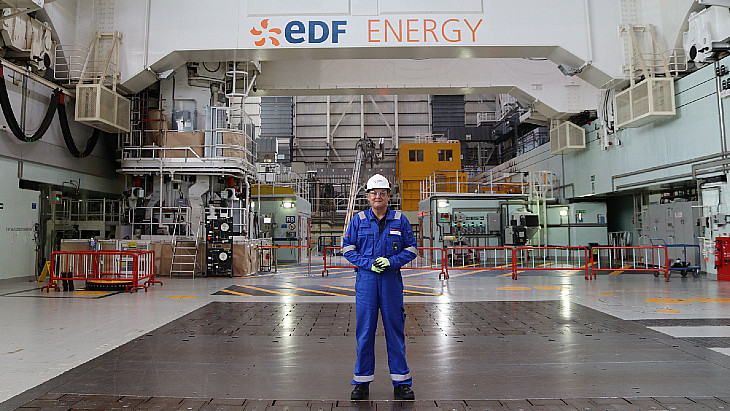
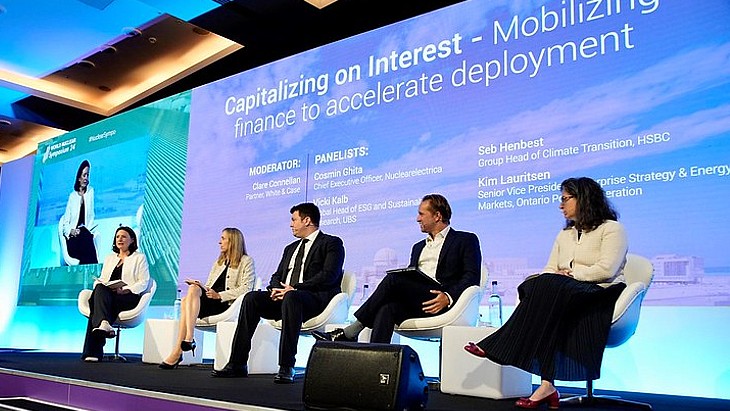
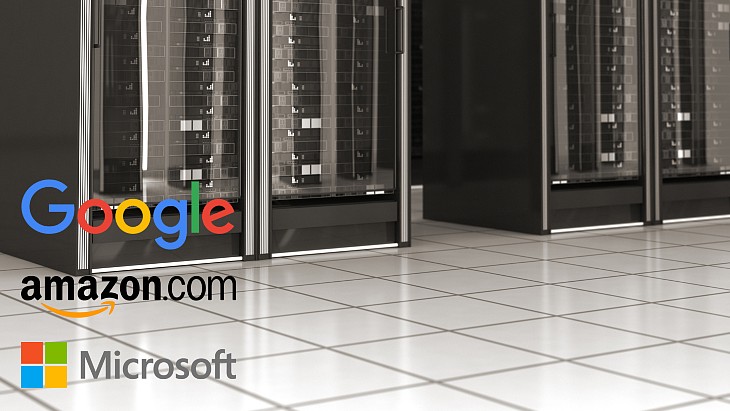
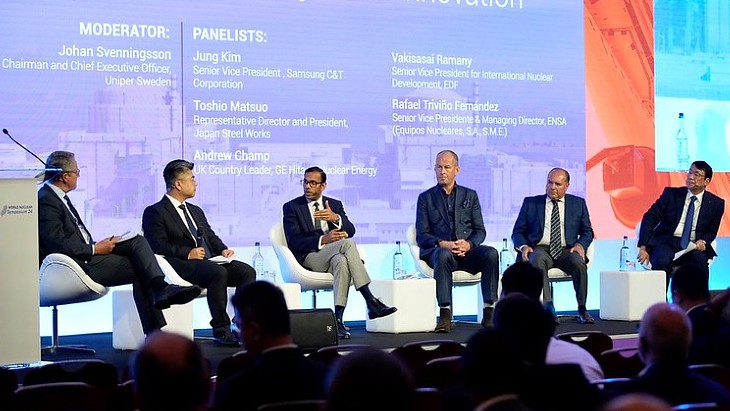
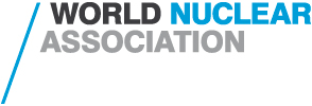